People Analytics Series (Part 4 of 4): Analysis of Data
- Kiwi Partners
- Sep 27, 2023
- 3 min read
Updated: Oct 30, 2023
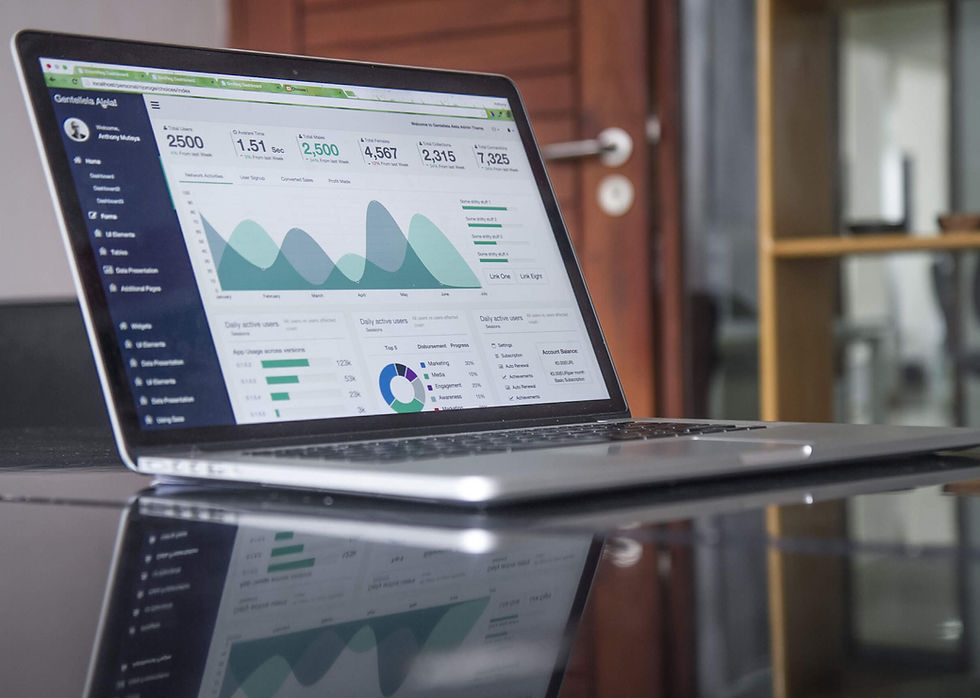
In previous articles, we have introduced people analytics and provided steps on how to prepare for taking data-driven action, and offered insights into the collection of data.
In the final segment of this series, we are going to provide tips on how to best analyze data once it has been gathered. To get started, here are some helpful questions to ask yourself:
Is the data accurate? Can it be trusted?
Is the data consistent? Does it line up with other systems?
Is the data meaningful?
These questions are designed to first ensure data integrity as well as determine whether or not coding needs to happen in order to link data between two different systems. Cleaning (e.g. correcting or removing incomplete or inaccurate data, eliminating duplicates, checking for outliers) then categorizing the data source(s) into codes or clusters will help facilitate analysis and allow us to draw better conclusions. Even qualitative and open-ended pieces of information can be assigned a code. For example, in looking at employee survey responses, you could manually classify feedback as Positive, Neutral, Negative and/or Management, Value, Leadership.
In order for data to have meaning, it should support the hypothesis we’ve asked ourselves. As mentioned in the preparation stage, a hypothesis is usually an if/then prediction about variables of interests. To expand on this further:
Variable: anything you are trying to measure (for example, satisfaction, engagement, retention, etc.).
Dependent variable: Output of a process that depends on or responds to other variables
Independent variable: Output to a process that influences other variables.
Example: My hypothesis is that if an employee is more engaged, then performance will increase. In this case, if the engagement (independent variable) increases, you would expect performance to change (dependent variable).
Once the data has value, the most objective approach would be to then apply statistical methods to analyze the data. You can take a look at the mode (most frequent data point), median (the middle value), and the mean (the sum of all values divided by the number of all values in the dataset). From there, you can determine if there is a correlation^ or a causation*.
^Correlation: A mathematical relationship that exists between two variables.
*Causation: One variable is the result of the occurrence of another variable (cause and effect).
Below are some tips to further help draw conclusions from the data:
Look for patterns
Convert data into visuals
Bring together multiple pieces of data and approaches to tell a better story.
For example, if you were trying to determine which recruiting channel was the best-performing in order to reduce your recruiting budget, it would be helpful to visualize several factors per channel, such as: Cost of 1st Year Turnover, Amount of 1st Year Turnover, and 1st Year Performance.
From these key findings, it is then up to you as a leader to develop recommendations and/or suggest specific interventions or strategies to address the issue(s). To allow the audience to better digest and feel vested, it is important to use the data to tell a story by using visuals as well as tapping into the business need and impact to their personal or professional goals.
What gets measured, gets done. Through tying timelines and key milestones with underlying data, an organization will only further support its accountability and growth.
If you have any questions, feedback, ideas or would like to continue the conversation, please contact the Kiwi Partners’ HR Services team.
Comments